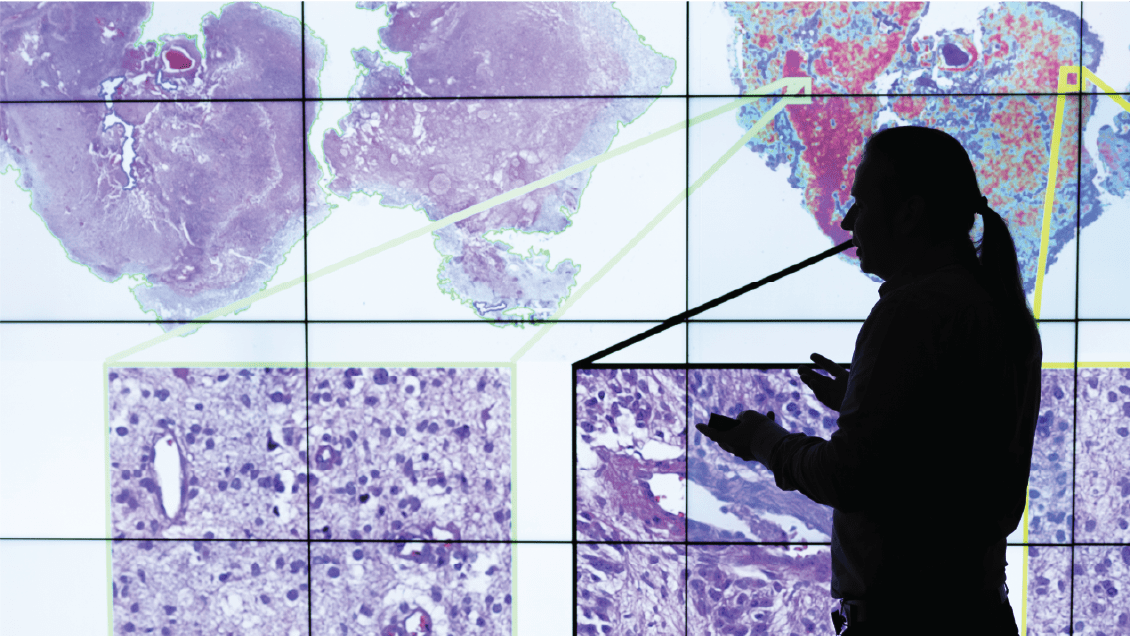
Spyridon Bakas, PhD, who came from the University of Pennsylvania a year ago, says IU School of Medicine can become a hub for a newer, decentralized approach to AI.
AI that Learns without Borders
Decentralized approaches to AI make it easier for scientists to share data, protect sensitive data and develop tools that reflect the diversity of patients.
Matthew Harris Dec 17, 2024
WHEN YOU’RE FIRING off a text message, you might notice your smartphone anticipates the next word or phrase you’re looking for.
That intuition is the result of a process called federated learning. And at IU School of Medicine, Spyridon Bakas, PhD, is deploying it to develop cutting-edge AI models that can detect the extent of brain tumors, quantify a person’s risk of breast cancer or even predict patient survival.
Seven years ago, when Bakas was at the University of Pennsylvania, he learned about this decentralized approach to AI while watching Google give a presentation at a statistical conference.
Back then, Google used it for a mundane objective: learning trends in language to improve the predictive texting at work on our smartphones each day.
Android phones came equipped with a machine-learning software model that was trained on raw data generated from a person typing out messages. When a user’s phone is charging overnight, that model is shipped back to the Google cloud. Data from billions of Android users enabled Google to create a consensus model.
Bakas saw this as a solution to a problem dogging his lab. His team was focused on building a library of brain imaging scans. Developers wanted to use them to train AI models to detect the extent of rare tumors like glioblastoma. Theoretically, clinicians would use the models to better target radiation therapy — sparing precious brain tissue while destroying cancer cells.
Federated learning offered a solution to two persistent problems. First, other institutions had privacy concerns about de-identifying patient data. And second, leery collaborators are protective of image collections they’ve curated for years.
So, Bakas’ team developed a model and shipped it to other institutions, who trained it on their brain images and sent the tweaked model back. “It’s private by design,” Bakas said. “Instead of putting data to the model, we send the model to the data.”
Where he had previously collected data of 2,000 patients in 10 years, Bakas and his collaborators this time collected 6,300 cases in 10 months. And they came from a much wider field — 71 institutions on six continents. The new model performed 33 percent better than traditional methods.
“It unlocked those silos,” he said.
Now, Bakas is an associate professor of pathology and laboratory medicine at IU School of Medicine. And he thinks the school can become a hub for this emerging field.
Recruited here a year ago, Bakas, who is the Joshua Edwards Scholar in Pathology & Laboratory Medicine, oversees the Center for Federated Learning for Medicine. It’s bringing together experts in fields like imaging, genomics, biostatistics and cyber security. His lab, including his entire staff that relocated from Penn to IU, made a quick impression. In August, the National Institutes of Health awarded Bakas’ team a $3.7 million grant to lead a multi-institutional effort to use federated learning to predict breast cancer risk.
Researchers frequently rely on small pools of patients, which are often heavily local. They might develop an AI model that spits out accurate predictions — for that sliver of people. Yet that accuracy can wane when used for a different group of patients. “The question isn’t only about increasing numbers,” Bakas said. “It comes in terms of diversity.”
In the IU-led project, five institutions will contribute advanced screening scans from women they treat. Those images act as a timeline for individuals. With enough clear scans, it’s reasonable to infer some women will never develop cancer. They will define normal breast tissue.
For women who develop breast cancer, prior scans serve as a vital contextual record, pointing perhaps to early warning signs of cancer. “That way,” Bakas said, “we can tell the likelihood of developing cancer five, six or 10 years in advance.”
The benefit goes beyond stratifying risk. Down the line, federated learning could be used to build models predicting which treatment regimen works best, allowing oncologists to finely tune care. Eventually, Bakas said, models using federated learning could have a similar impact on other cancers.
“They get the appropriate treatment and avoid the potential toxicity of therapy they don’t need,” Bakas said. “That improves quality of life.”
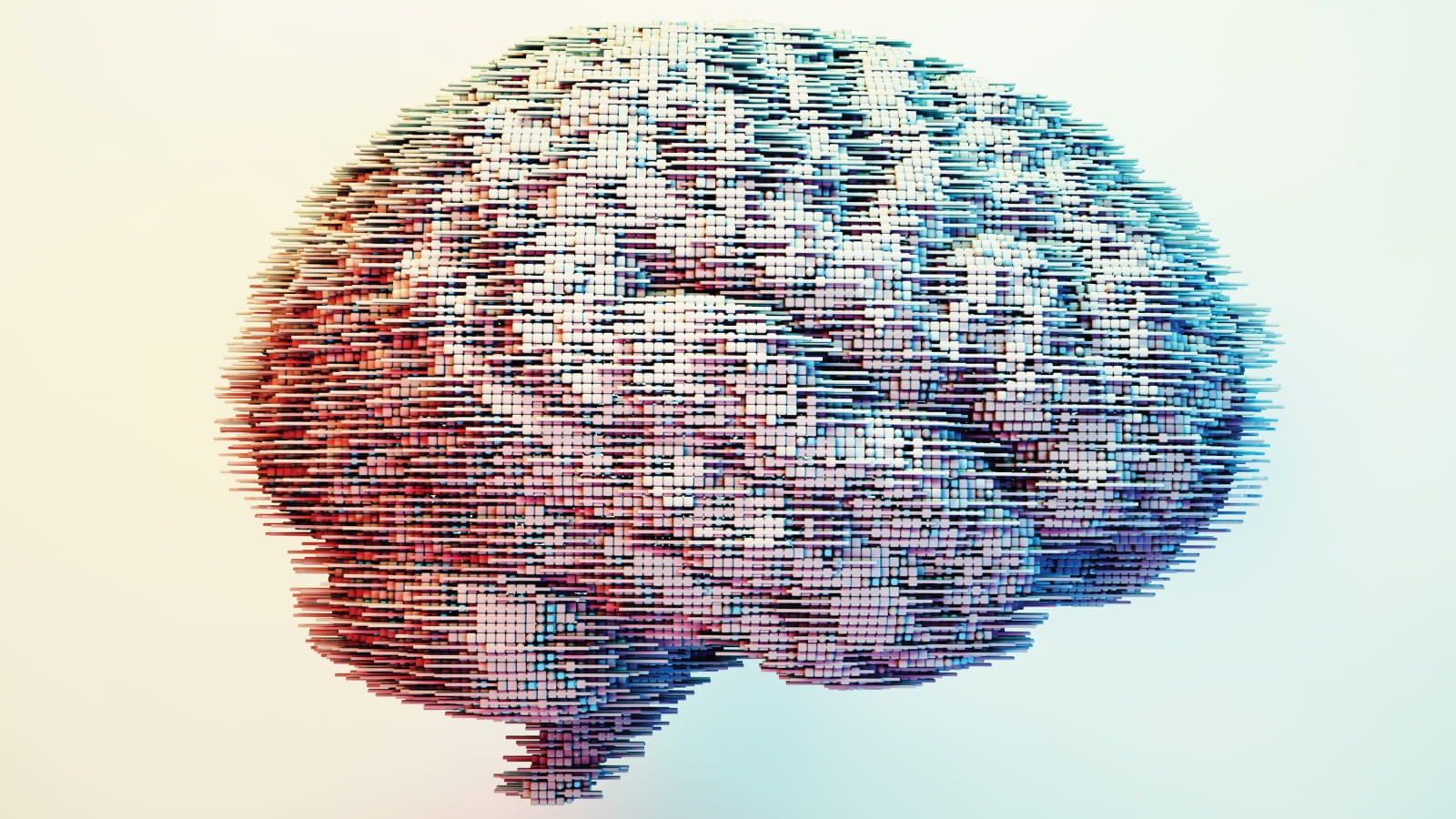
Unlocking the Power of AI
IU School of Medicine embraces a powerful new tool to speed research and treat patients.
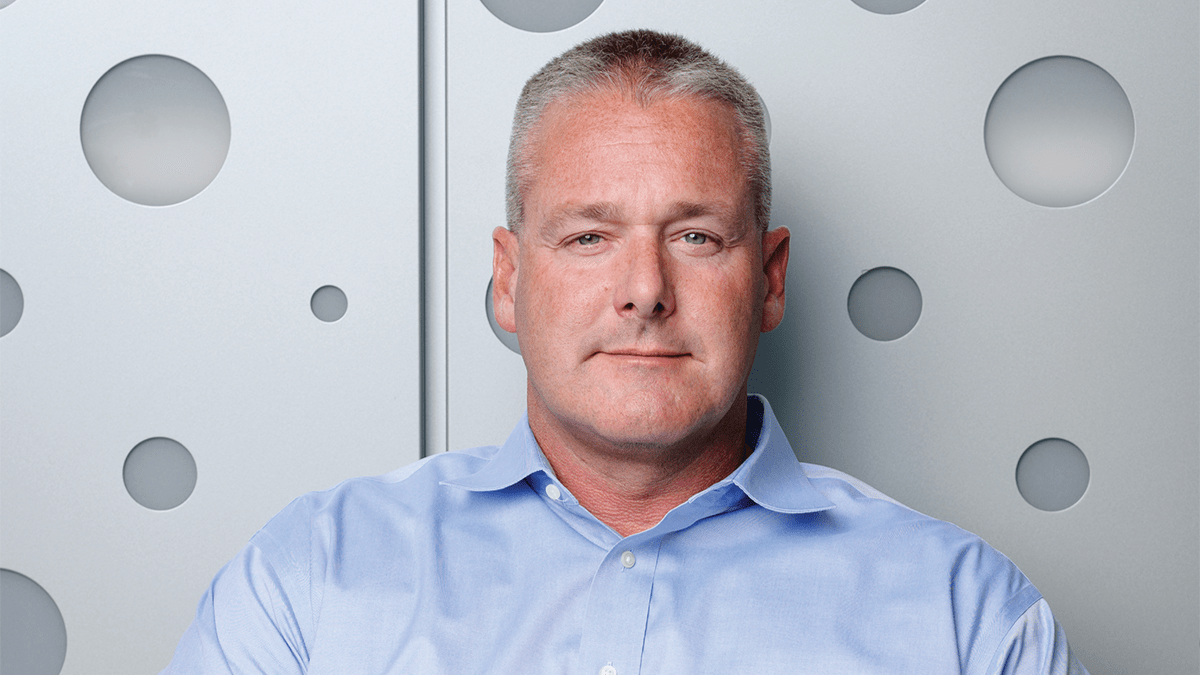
How Radiology is Becoming a Leader in Adopting AI
Few clinical areas have adopted AI tools faster than radiology, easing workloads and helping overcome a shortage of clinicians.
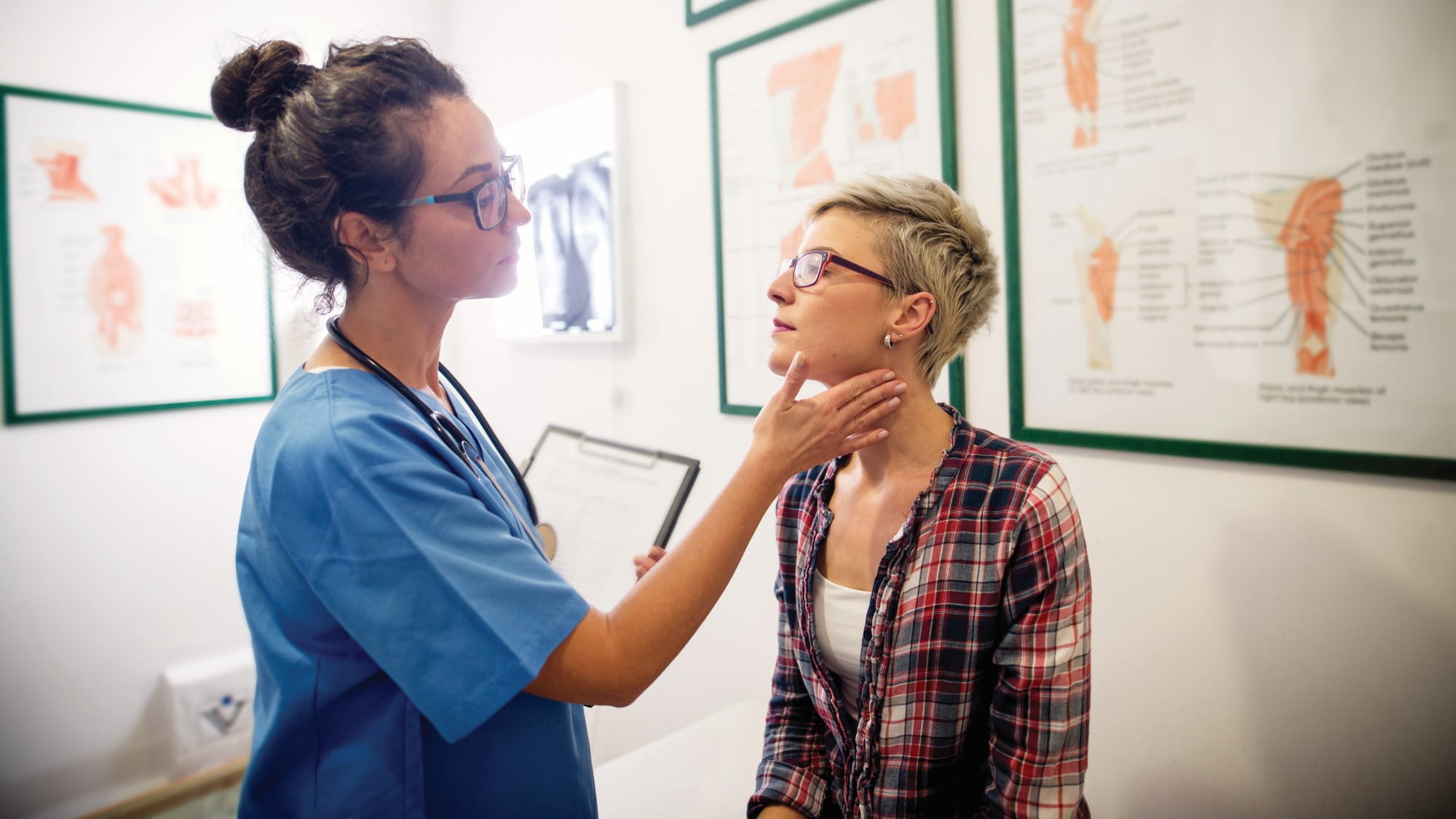
AI is Coming to an Exam Room Near You
Ambient listening interprets conversations in real-time to update electronic health records — and help physicians reconnect with their patients.
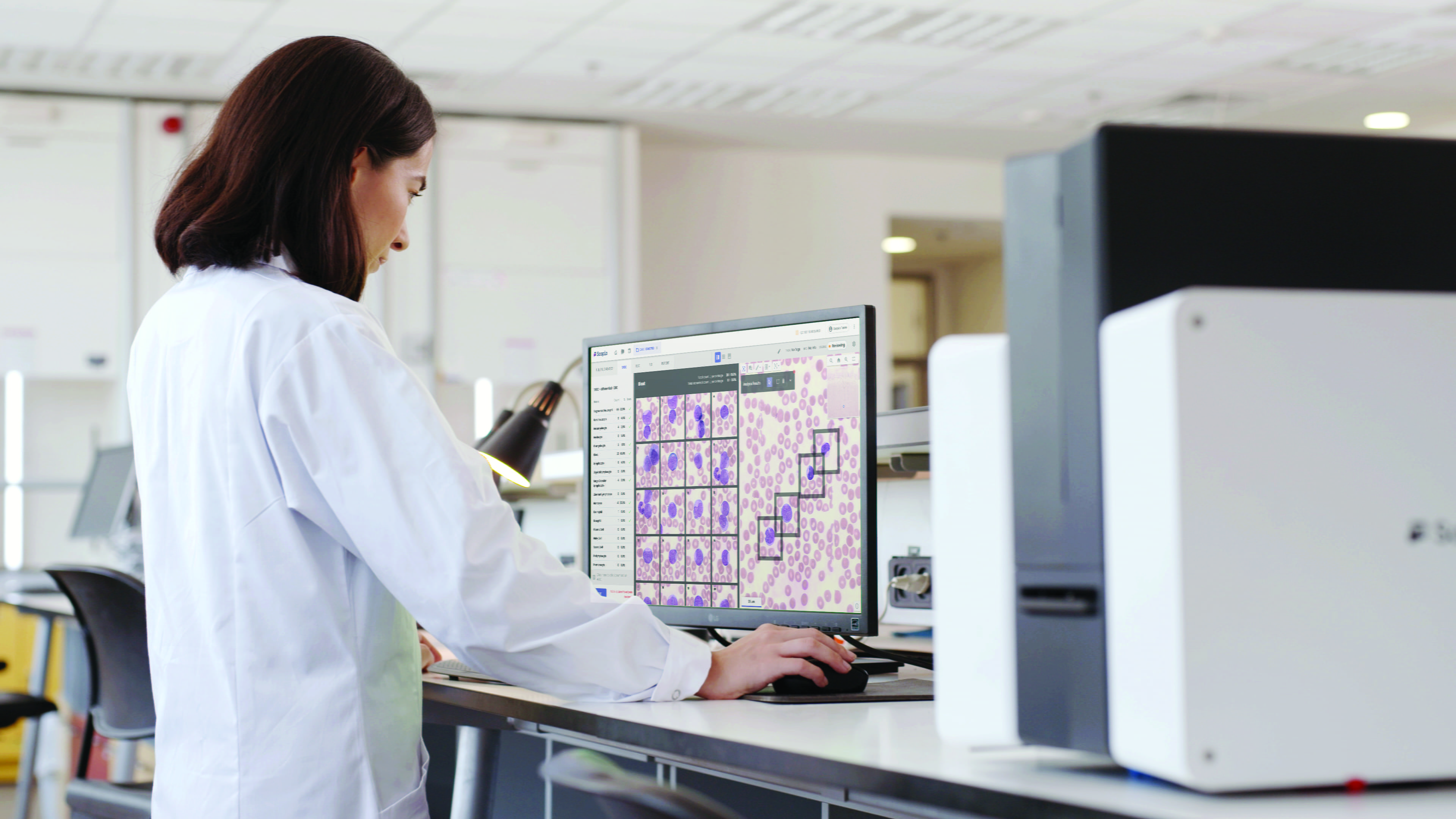
"We're on the Precipice"
AI powers tools that help pathologists spend less time counting cells and use their refined skills to make complicated diagnoses.
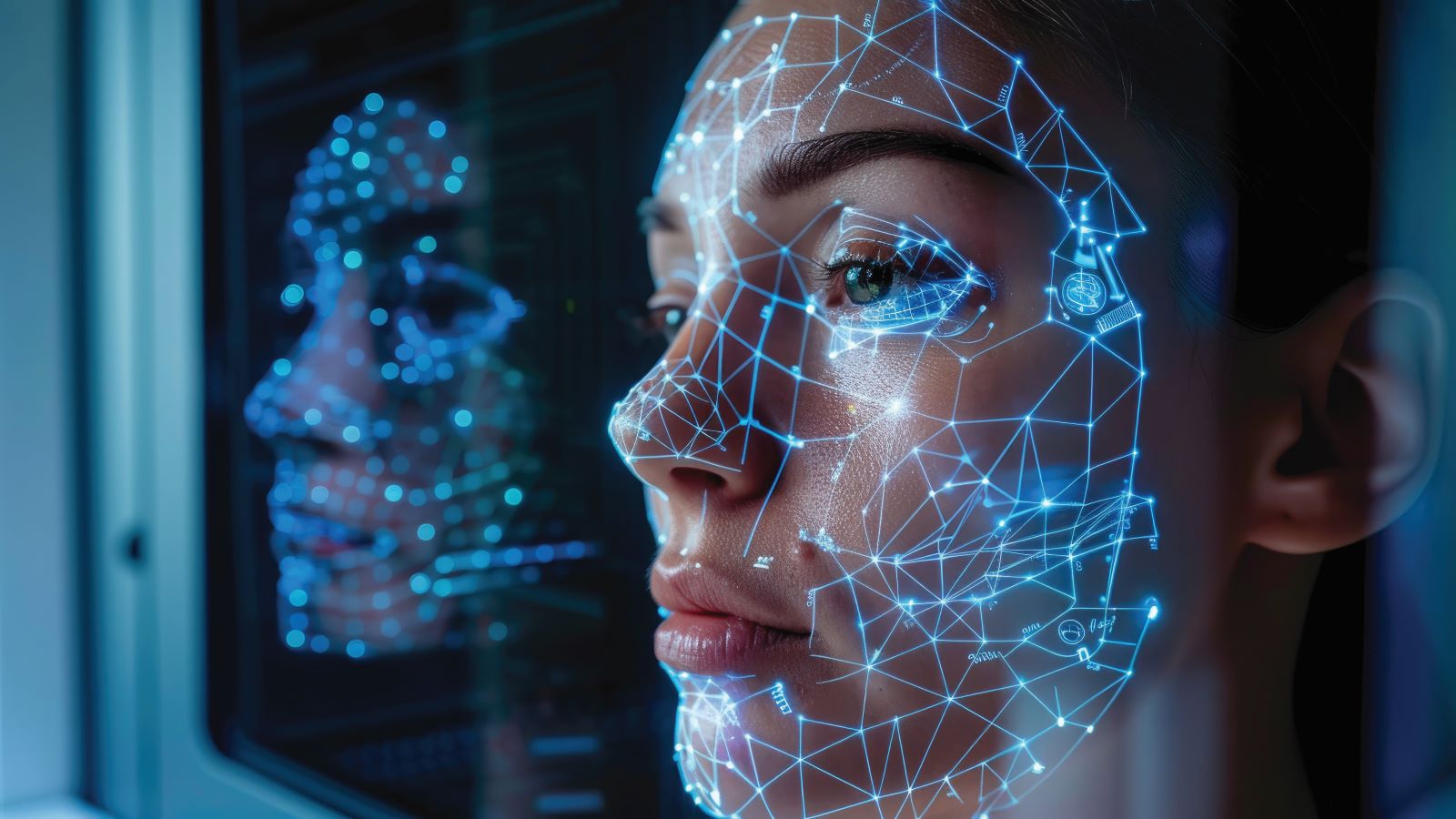
Skin in the Game
An IU researcher’s AI tool shows promise in predicting whether melanoma will return.
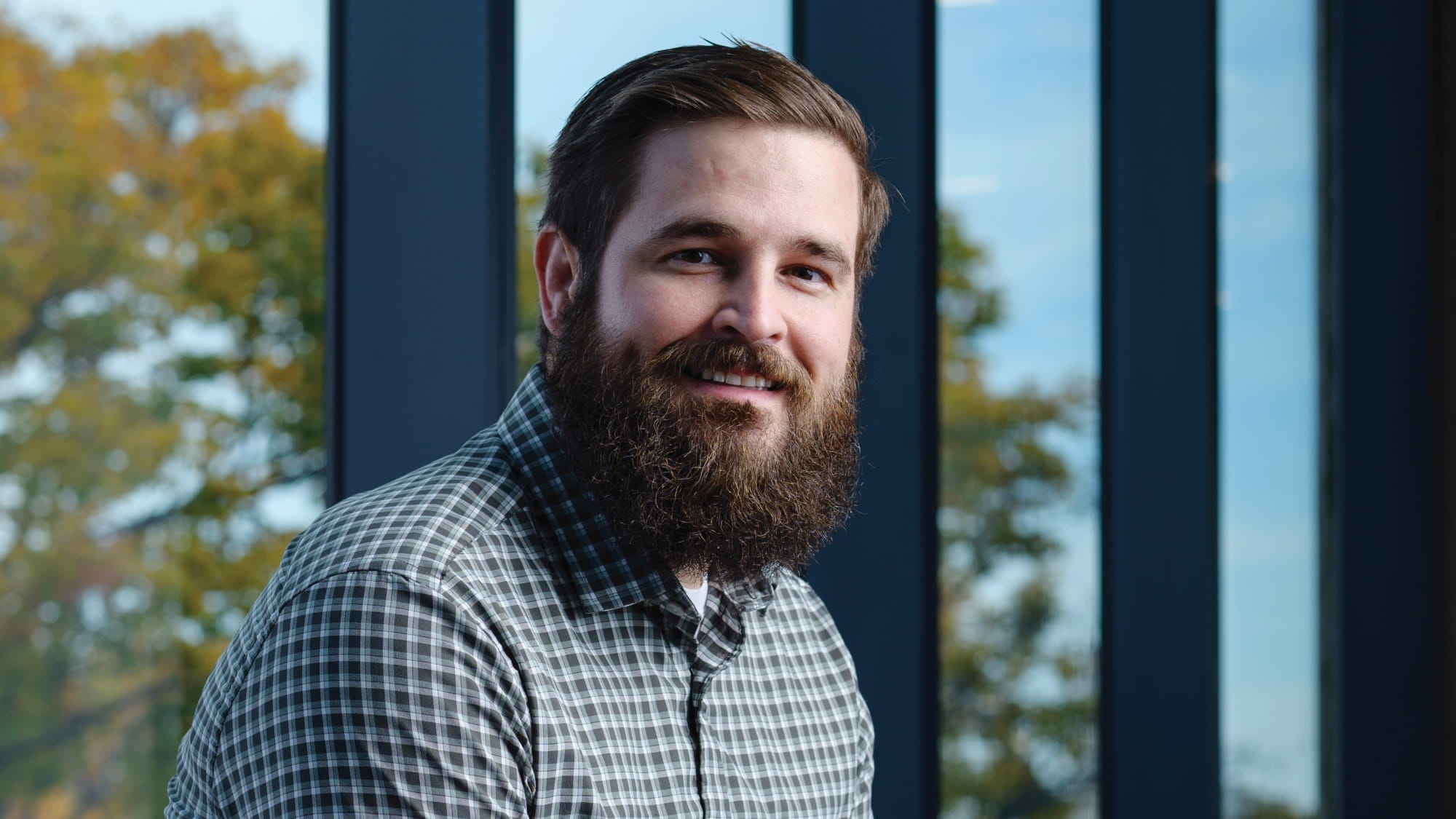
Finding a Signal in Noise
Machine learning enables cancer researchers to sift reams of genetic data and identify a protein potentially powering multiple myeloma.
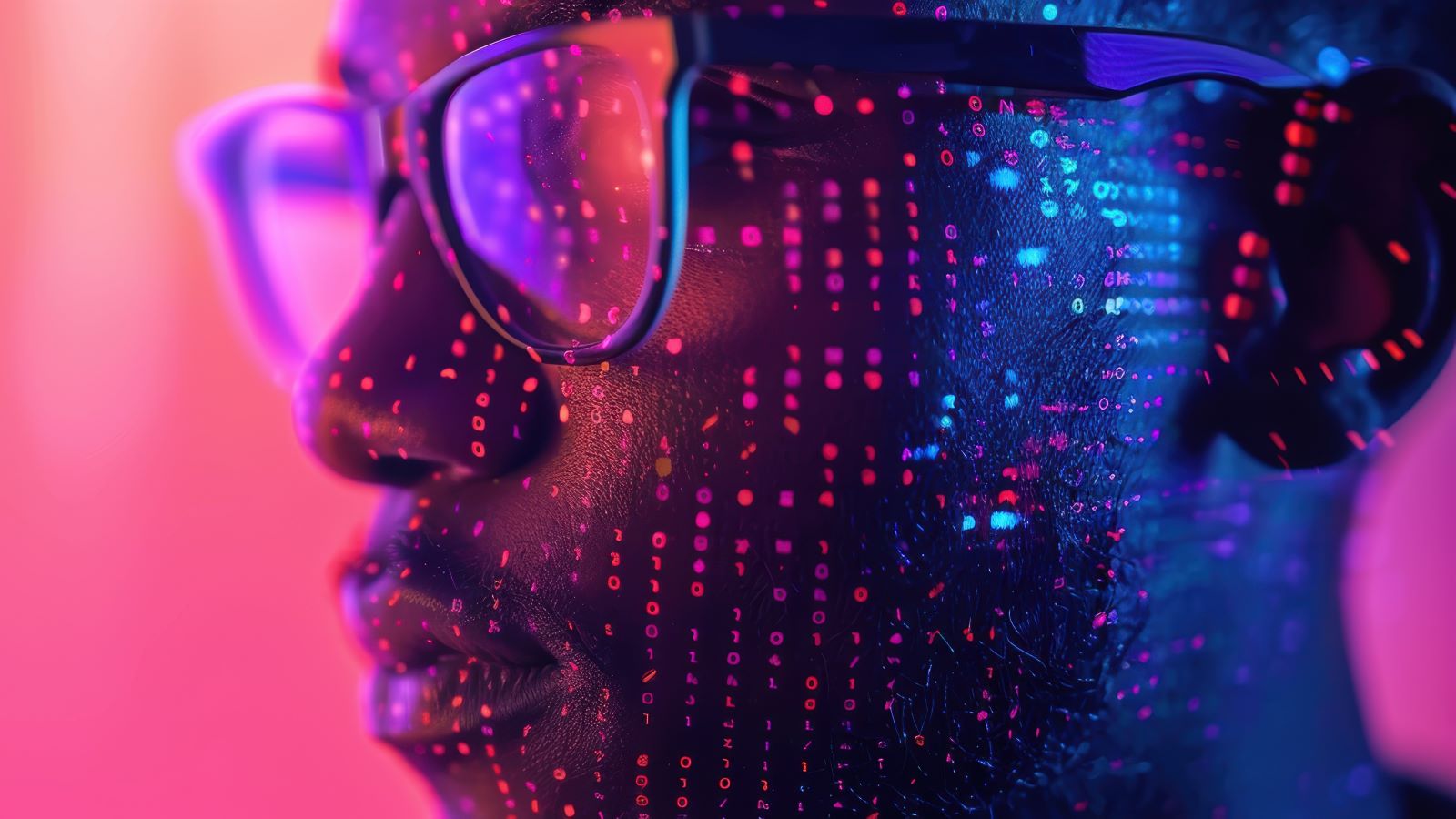
Reducing Bias in AI
In a technology hungry for data, how do we ensure it consumes good information and builds models that benefit every patient?