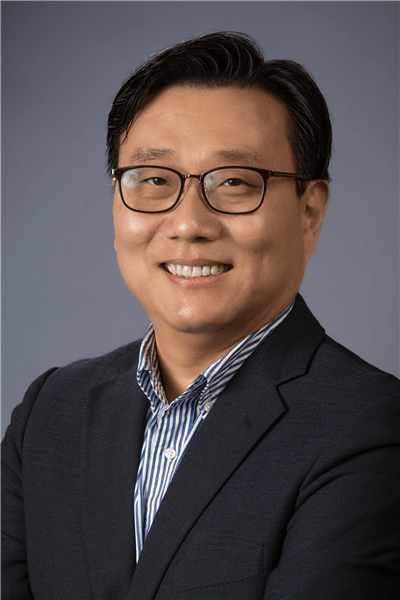
Taeho Jo, PhD
Assistant Professor of Radiology & Imaging Sciences
- Phone
- 317-963-7504
- Address
-
355 W. 16th Street, GH 4093
Indianapolis, IN 46202
Bio
My research focuses on using artificial intelligence and deep learning to advance our understanding of Alzheimer's disease. With a background in biochemistry, biophysics, neuroinformatics, and deep learning, I have developed innovative models to help detect and classify Alzheimer's disease at an early stage using brain imaging data. For example, I created a 3D convolutional neural network (CNN) model for tau PET scans, which has improved the accuracy of Alzheimer's diagnosis.
In addition to imaging, my research includes studying genetic variants linked to Alzheimer's and developing methods to analyze lipidome data. These efforts help predict how the disease might progress from Mild Cognitive Impairment to Alzheimer's.
Supported by the Alzheimer's Association, I am dedicated to making my research accessible and useful to the broader community. I focus on creating user-friendly tools and sharing the source code for my projects to encourage further advancements in Alzheimer's research.
Year | Degree | Institution |
---|---|---|
2010 | PhD | Tokyo Medical and Dental University |
2002 | BA | Inha University |